101 Series | Asset Security| Information Life cycle
Beyond acquisition, use, archival and disposal
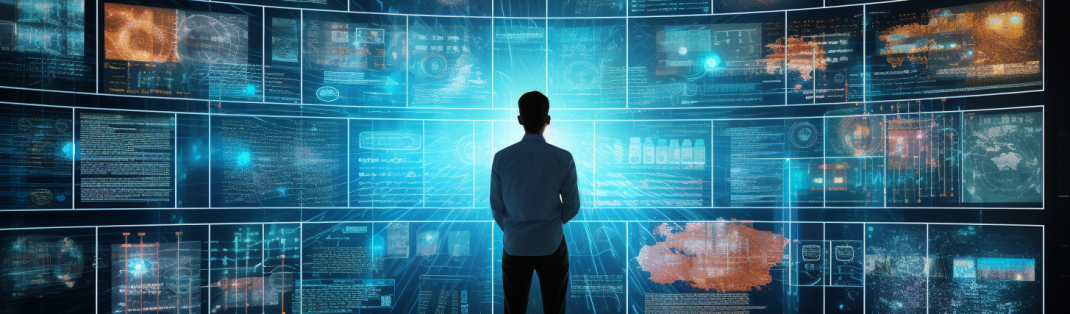
In today's data-driven world, the need to know about how data is being created, managed and disposed become hugely important. Probably here we should mention that Understanding this cycle helps organisations manage their data effectively and make informed decisions, but we are going to go beyond that: Everybody should Understand this cycle to make informed decisions.
Yes, again this is a data-driven world, so not just organisations, all kinds of people must be aware of this. Maybe in the near future there will exist an Elementary school course (this is a very serious suggestion we are making here).
So let's dive deep into the matter. Firstly know the significance in different industries:
In the
business
sector, the information lifecycle plays a critical role in decision-making, customer relationship management, and overall operational efficiency. Businesses rely on accurate and timely data to identify trends, analyse customer preferences, and optimise their strategies.
Example: Retail Analytics
A retail company collects sales data from various sources, including online platforms and physical stores.
In
healthcare, the information lifecycle ensures patient data is securely managed and shared among medical professionals while adhering to privacy regulations. Effective information management supports accurate diagnoses, treatment planning, and medical research.
Example: Electronic Health Records (EHR)
Healthcare providers maintain electronic health records for patients. These records include medical history, test results, and treatment plans.
The
education
sector benefits from the information lifecycle by enabling personalised learning experiences, tracking student progress, and enhancing administrative processes. Educational institutions manage student records and academic data from admission to graduation.
Example: Learning Analytics
In educational technology, learning analytics involves collecting and analysing student data to understand learning patterns.
But again, what about the date we generate, store, share, (and sometimes forget to delete) as an individual?
For more information in regards Individual Information Lifecycle, we suggest the follow article:
Personal Information Series | Individual Information Life cycle.
Ok let's jump to yet another important topic: Stages of the Information Lifecycle. In this section, we wanted to go a little bit further than Acquisition, Use, Archival and disposal.
Acquisition
(either copied from elsewhere or created):
1. Data Generation and Capture: generation of data through various means, such as user inputs, sensors, devices, applications, and automated processes.
2. Context and Metadata: Contextual information includes details like who created the data, where and when it was generated, and the purpose behind its creation.
3. Accuracy and Completeness: Ensuring that the data entered or captured is accurate and complete helps prevent errors and inconsistencies.
4. User Inputs and Engagement: In platforms where user-generated content is prevalent, such as social media, the creation phase involves users actively participating by posting text, images, videos, comments, and more.
5. Automation and Integration: Automation technologies, such as IoT devices and sensors, could play a significant role in the creation phase.
6. Importance of Timeliness: Real-time data feeds from social media, financial markets, or weather sensors provide immediate insights.
Storage and Organization: in regards to how information is stored securely and organised for easy retrieval: databases, cloud storage, and physical archives. This phase involves securely storing data in a structured manner, making it accessible for future retrieval and analysis.
1. Data Storage Solutions: here you select appropriate storage solutions based on factors like data volume, type, security requirements, and accessibility.
2. Structured and Unstructured Data: we can categorise into structured, unstructured, or semi-structured formats. Structured data fits neatly into rows and columns, like data in databases. Unstructured data, such as text and multimedia, lacks a predefined structure. Semi-structured data includes metadata and can be organised flexibly.
3. Data Categorization and Indexing: label data to facilitate quick retrieval and analysis. This involves tagging data with relevant keywords, assigning categories, and creating indexes that act as signposts to locate specific information efficiently.
4. Data Management Systems: DBMS play a critical role in organising structured data. These systems allow data to be stored, retrieved, updated, and managed using standardised techniques. They ensure data integrity, security, and enforce access controls.
5. Backup and Redundancy: prevent data loss due to hardware failures, cyberattacks, or other unforeseen incidents. Regular backups ensure that data can be restored to a previous state if needed.
6. Access Control and Security: Data should be accessible only to authorised users, and encryption techniques can be employed to protect sensitive information.
Processing and Analysis (also called Use stage or step): now we are going to discuss the role of processing in transforming raw data into actionable knowledge.
1. Data Preparation: this involves handling missing values, removing duplicates, and converting data into a standardised format.
2. Data Processing Technologies: these platforms allow for the efficient handling of large volumes of data and support complex processing tasks.
3. Security Controls: our passion now to be discussed. Several security considerations to apply during this phase:
Access Control: Use role-based access mechanisms to limit permissions based on users' roles and responsibilities.- Data Encryption: Apply encryption techniques to sensitive data both at rest and during transit.
- Data Masking: Mask sensitive data during analysis so that the actual data remains concealed while still allowing valid insights to be drawn. This is particularly useful when sharing data with third parties or conducting tests.
- Anonymization: When sharing data for research or collaboration, anonymize personal information to protect privacy. This involves removing or altering identifiers that could link the data back to individuals.
- Audit Trails and Logging: Maintain comprehensive audit trails and logs of data processing activities. This helps track who accessed the data, what changes were made, and when these actions occurred.
- Data Minimization: Only process and analyse the data necessary for the specific task. Minimising the amount of sensitive data in use reduces the potential impact of a security breach.
- Secure Processing Environments: Ensure that the environments where data is processed are secure. This includes using trusted tools and software, regularly updating systems, and implementing intrusion detection systems.
Distribution and Sharing: involves disseminating data and insights to relevant stakeholders within and outside an organisation. This phase is crucial for effective communication, collaboration, and decision-making.
1. Collaboration Tools: email, messaging platforms, project management software, and cloud-based document sharing platforms.
2. Audience Segmentation: It's important to segment your audience and tailor the distribution of information based on relevance and need-to-know.
3. Secure Sharing Methods: Encrypted email attachments, secure file-sharing platforms, and password-protected documents are examples of measures to protect data during transit.
4. Data Privacy and Consent: Ensure that individuals are aware of how their data will be used and who will have access to it.
5. Transparency and Accountability: Being transparent about the source, accuracy, and potential limitations of the data promotes trust and accountability.
Archiving and Retention: Let's explore this phase in detail, with an emphasis on the differences between archiving and backup:
1. Archiving: refers to the process of moving data that is no longer actively used to a separate storage location for long-term preservation. Key aspects:
Purpose: Archiving is primarily intended for historical reference, compliance, and potential future use.- Retrieval: Archived data is typically stored in an organised and searchable manner, making it easier to retrieve when needed.
- Retention Periods: Archiving involves defining retention periods based on legal and regulatory requirements.
- Data Format: Archived data is often stored in formats that are both durable and independent of specific software versions, ensuring that the data remains accessible even as technology evolves.
2. Backup: Backup involves creating copies of data to protect against data loss due to hardware failures, human errors, or other unforeseen incidents.
Retrieval: Backups are designed for quick data recovery in case of emergencies. They prioritise speed of retrieval over organisation and historical reference.- Retention Periods: Backup retention periods are generally shorter than those for archives. Backups are kept for a period that allows for quick recovery of recent data, and older backups are often replaced with newer ones.
- Backups often mirror the format of the active data, as the primary purpose is to restore the data to its original state.
Differences between Archiving and Backup: Purpose: Archiving focuses on preserving historical and valuable data for long-term reference, compliance, and legal reasons. Backup is focused on creating copies for data recovery in case of emergencies or data loss.
Disposal and Deletion: Proper data disposal is crucial to maintain data security, compliance with regulations, and to prevent unauthorised access to sensitive information.
1. Data Classification: Before disposal, it's important to classify data based on its sensitivity, legal requirements, and business value.
2. Regulatory Compliance: Different industries and regions have specific requirements for data destruction, such as the General Data Protection Regulation (GDPR) or the Health Insurance Portability and Accountability Act (HIPAA).
3. Secure Erasure: Sensitive data should be securely erased using proper methods that render the data unrecoverable. This can involve overwriting the data with random characters multiple times or using specialised software designed for secure data destruction.
4. Physical Destruction: For physical media like hard drives, CDs, or tapes, physical destruction might be necessary. This can involve shredding hard drives or incinerating optical discs to ensure data cannot be retrieved.
5. Document Shredding: For paper documents, shredding is a common method of disposal. Shredded paper is difficult to reconstruct, reducing the risk of information leakage.
6. Digital Media Disposal: For digital devices like smartphones, tablets, or USB drives, ensure data is completely wiped before disposal. Many devices offer a "factory reset" option, but additional steps might be needed to ensure complete data removal.
7. Decommissioning Systems: When retiring IT systems or hardware, ensure data is properly removed from them before disposal. This might involve formatting drives, removing storage media, and securely wiping system memory.
Long reading, right? Well we tried to shorten as much as possible. Now you know that organisations should not be the only entities to understand and master these concepts. We encourage you to potentialize this awareness by implementing these concepts into your daily life as well at your organisation.
Source and further reading.
Shahbaznezhad, H., Dolan, R., & Rashidirad, M. (2021). The role of social media content format and platform in users’ engagement behavior.
Journal of Interactive Marketing,
53, 47–65.
https://doi.org/10.1016/j.intmar.2020.05.001
Farmer, D. (2021). 6 top business benefits of real-time data analytics.
Business Analytics.
https://www.techtarget.com/searchbusinessanalytics/feature/6-top-business-benefits-of-real-time-data-analytics
Harris, Shon & Maymí, Fernando. CISSP EXAM GUIDE Seventh Edition. New York McGraw Hill Education, 2016.